Learn to Compress & Compress to Learn
Workshop at the International Symposium on Information Theory (ISIT) 2025
In parallel, compression has emerged as a powerful proxy task for advancing broader learning objectives, including representation learning and model efficiency. Recent research is exploring how compression can enhance training and generalization of large-scale foundation models for vision, language, and multi-modal applications. Techniques like knowledge distillation, model pruning, and quantization share common challenges with compression, highlighting the symbiotic relationship between these seemingly distant concepts. The intersection of learning, compression and information theory offers exciting new avenues for advancing both practical compression techniques and also our understanding of deep learning dynamics.
This workshop aims to unite experts from machine learning, computer science, and information theory to delve into the dual themes of learning-based compression and using compression as a tool for learning tasks. We are excited to feature industry experts who will share valuable practical insights with the ISIT attendees.
The workshop was be held on Thursday 26 June 2025 in Ann Arbor, it was great to see you all!
🏆 The winner of the best paper award is: Online Conformal Compression for Zero-Delay Communication with Distortion Guarantees by Unnikrishnan Kunnath Ganesan, Giuseppe Durisi, Matteo Zecchin, Petar Popovski and Osvaldo Simeone. Congratulations!
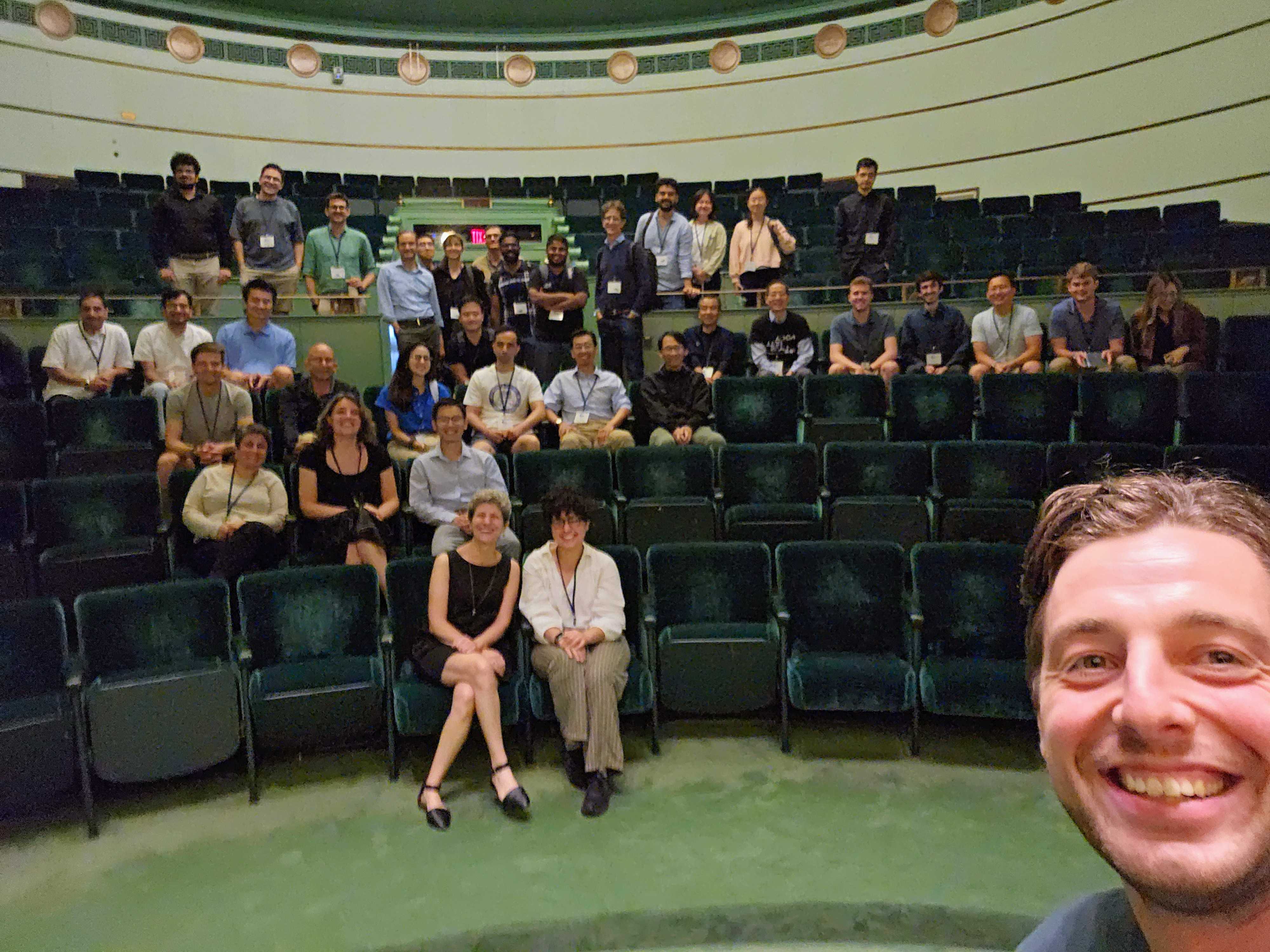
Location
The workshop will be held on Thursday 26 June 2025 on the 4th floor of the the Rackham Graduate School, 915 E Washington St, Ann Arbor, MI 48109, USA. See the venue on Google Maps, and the floorplan below.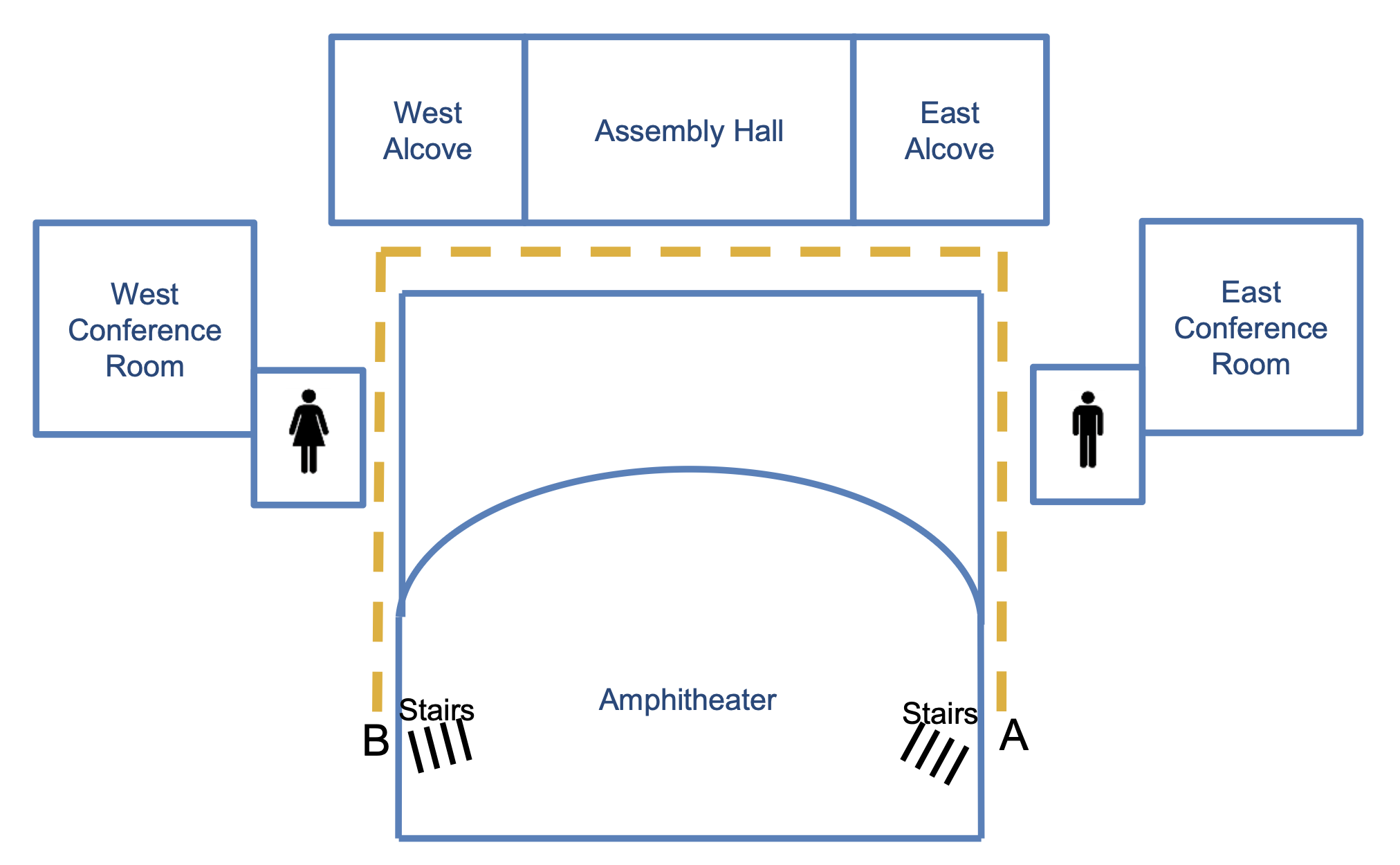
Accepted Posters
Spotlight papers (in alphabetical order):-
Discretized Approximate Ancestral Sampling
Alfredo De la Fuente, Saurabh Singh and Jona Ballé -
DeCompress: Denoising via Neural Compression
Ali Zafari, Xi Chen and Shirin Jalali -
Online Conformal Compression for Zero-Delay Communication with Distortion Guarantees
Unnikrishnan Kunnath Ganesan, Giuseppe Durisi, Matteo Zecchin, Petar Popovski and Osvaldo Simeone
Important Dates
- Paper submission deadline:
March 14March 28, 2025 (11:59 PM, anywhere in the world!). - Decision notification: April 18, 2025
- Camera-ready paper deadline: May 1, 2025
- Workshop date: June 26, 2025
Invited Speakers
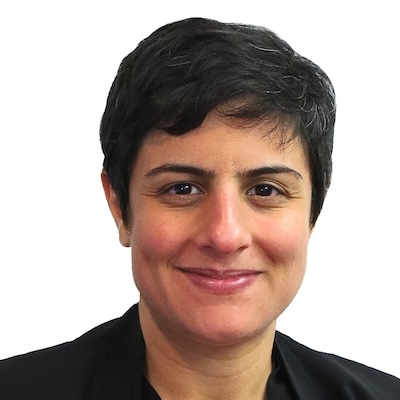
Prof. Shirin Saeedi Bidokhti
University of Pennsylvania
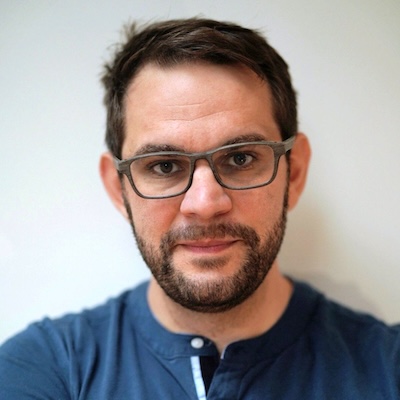
Prof. Ferenc Huszár
University of Cambridge
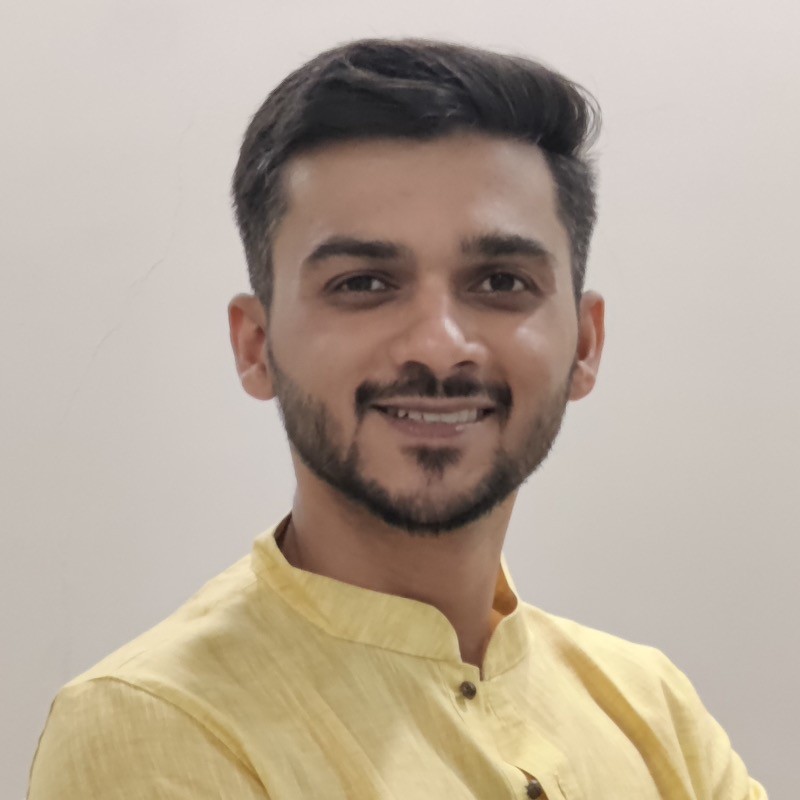
Dr. Pulkit Tandon
Granica.ai
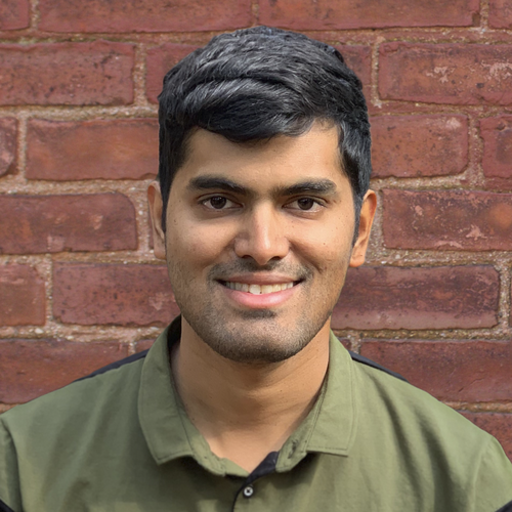
Dr. Kedar Tatwawadi
Apple
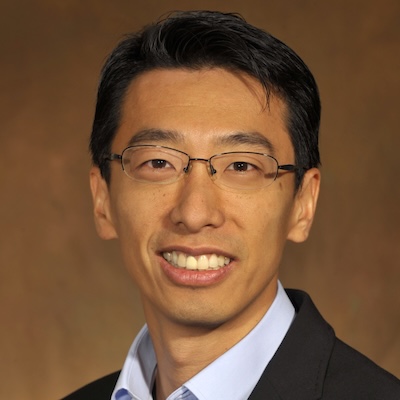
Prof. Chao Tian
Texas A&M University
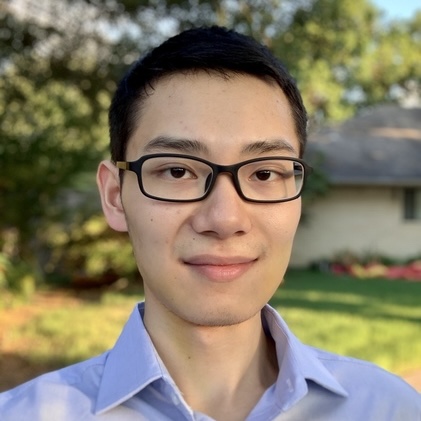
Dr. Yibo Yang
Chan Zuckerberg Initiative
Organizers
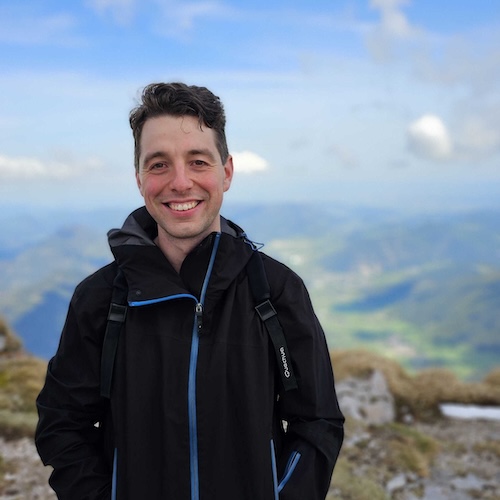
Gergely Flamich
University of Cambridge / Imperial College London
Ezgi Ozyilkan
New York University
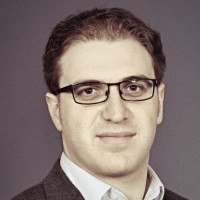
Prof. Deniz Gündüz
Imperial College London
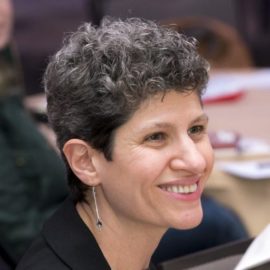